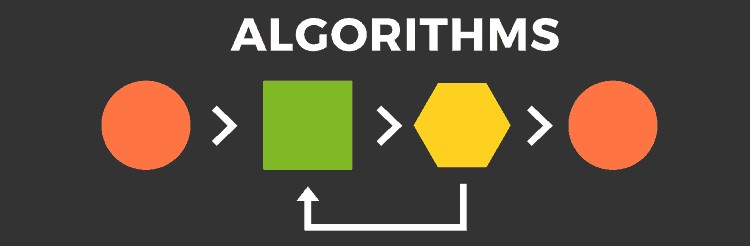
Local Differential Privacy
This is an algorithm that will ensure the privacy of the users data before it is sent across to the linodes server.The way this algorithm works is that it will randomise the data that will be sent across 30% of the time. The remaining 70% of the time it is sending across the actual data.
Because the data is randomised before it is even sent across, this means that the server never sees or receives raw data. This means that even if someone manages to hack into the server where all the data is stored, they will not be able to learn too much about the user’s personal data.
On top of using Local Differential Privacy, we will also be only sending across the outbound postcode, which will make identifying the user even harder.
Prediction Algorithms of Wellbeing Score
The algorithm of calculating the wellbeing score is the average of ratio of actual steps/calls to the target steps/calls with an enlargement to scale from 0 to 10Wellbeing Score = [ (actual steps / target steps) + (actual calls / target calls) ] / 2 * 10
Our current app uses the above algorithm. However, this is not the most accurate form of calculating the well-being score. Therefore, in the next part of the project, the data that is sent to the linode server i.e. the number of calls, steps and well-being error rate (the well-being calculated by the app vs the well-being score inputted by the user), will be fed into a machine learning algorithm to come up with a better algorithm to calculate the well-being score.