Unit Testing
-
FISECARE
Testing Strategy
We conducted unit testing manually because it is more suited for testing a User Interface. With manual testing, we can review the look and feel of the interface and evaluate the user experience ourselves which requires subjectiveness that automated testing cannot provide. Furthermore, with manual testing, it is a lot easier to switch and make changes to the design because we can pinpoint design faults as we test.
Results
Test Case Pass/Fail Buttons are clickable Pass Websites are accessible Pass Websites can be easily navigated Pass Navigation is correct (e.g. back button should navigate to the previous page) Pass Websites are scaled correctly on the page and are viewable Pass -
MotionInput - Calibration
Testing Strategy
We have conducted several tests at different angles and positions of the camera to ensure the model is being stored under the correct file paths and the files are being saved. Then, MotionInput should be able to open and load the model from the correct file paths and predict the cursor’s coordinates. To test this storing and loading of files, we calibrated with different modes and ran MotionInput accordingly to ensure the program runs with no file handling errors.
Results
Test Case Pass/Fail Files are stored under the correct file paths for each model. Pass Whilst MotionInput is running, the model is loaded from the correct file. Pass Whilst MotionInput is running, loaded model is able to run predictions with the landmark’s coordinates. Pass -
MotionInput - Navigation
Testing Strategy
Again, with manual testing, we were able to test the gestures of each of our modes. For a similar reason to FISECARE, with manual testing, we were able to understand where the user may struggle with making gestures or which gestures would be easy sitting at an angle from the camera. This way we can be selective about what gestures we do use for our modes. For example, the open-mouth gesture which was initially used for left-clicking can be sensitive and cause unwanted clicking, especially from an angle, which can be frustrating. Therefore, we replaced this gesture event with smiling which resulted in better performance.
Results
Test Case deg=0 deg=30 deg=45 deg=60 deg=75 In head_joystick mode, turning head triggers cursor to move to intended locations on screen Pass Pass Pass Pass Pass In nose_tracking mode, turning head triggers cursor to move to intended locations on screen Pass Pass Pass Pass Pass In hand_tracking mode, moving left hand triggers cursor to move to intended locations on screen Pass Pass Pass Pass Pass In hand_tracking mode, moving right hand triggers cursor to move to intended locations on screen Pass Pass Pass Pass Pass Opening the mouth triggers the cursor to right-click. Pass Pass Pass Pass Pass Smiling triggers the cursor to left-click Pass Pass Pass Pass Pass Making a fish face triggers the cursor to double click Fail Fail Fail Fail Fail Opening mouth and looking down with trigger scrolling down Pass Pass Pass Pass Fail Opening mouth and looking up will trigger scrolling up Pass Pass Pass Pass Fail Speech commands are responsive and help to interact with the system effectively. Pass Pass Pass Pass Pass Pinching left index finger and thumb together triggers the cursor to left-click Pass Pass Pass Pass Pass Pinching right index finger and thumb together triggers the cursor to left-click Pass Pass Pass Pass Pass Pinching left middle finger and thumb together triggers the cursor to right-click Pass Pass Pass Pass Pass Pinching right middle finger and thumb together triggers the cursor to right-click Pass Pass Pass Pass Pass Holding up the middle and index finger using left hand will trigger scrolling up Pass Pass Pass Pass Pass Holding up the middle and index finger whilst curving fingers down will trigger scrolling down Pass Pass Pass Pass Pass Holding up the middle and index finger using right hand will trigger scrolling up Pass Pass Pass Pass Pass Holding up the middle and index finger whilst curving fingers down will trigger scrolling down Pass Pass Pass Pass Pass Making a fist using left hand triggers double clicking Pass Pass Pass Pass Pass Making a fist using right hand triggers double clicking Pass Pass Pass Pass Pass
Overall, it was found that hand mode provides better control over the system than face mode. Although, with Face Joystick mode it was a lot easier navigating using the face rather than with the Nose Tracking mode. However, the sensitivity of face gestures made it difficult to have complete control over interactions with the system due to the gestures’ unpredictability. Sometimes unwanted clicking will be triggered or there will be no clicking. Though these issues only seem prevalent when we reach extreme angles from the camera and when there is poor lighting, therefore, we can take these observations into account when choosing a suitable position for the camera.
Integration Testing
Testing Strategy
Integration testing is particularly important to ensure our bedside navigator is fully functioning and successfully achieves its goals of running FISECARE with MotionInput.
To test the capabilities of our modes and the suitability of the websites chosen for our extension of FISECARE we incorporated the use of MotionInput’s Bedside Navigator mode with FISECARE. Therefore, for each of the websites we gave each mode a rating out of 5, 1 suggesting that it was difficult navigating the website with the mode and 5 being it was easy and comfortable navigating the website with the mode.
Results
Website | Nose Tracking Mode | Joystick Mode | Hand Tracking Mode |
---|---|---|---|
Paint | 3 | 4 | 5 |
Calculator | 4 | 5 | 5 |
Scrabble | 2 | 3 | 4 |
Reading Books | 4 | 5 | 5 |
UNO | 3 | 4 | 4 |
Scratch Coding | 2 | 2 | 4 |
Studying | 3 | 5 | 5 |
Word Ladder Game | 3 | 5 | 5 |
Spotify | 3 | 4 | 5 |
Most websites are easy to navigate. However, for websites where smaller buttons present, users may struggle to click the button. A workaround for this problem would be to use the ‘zoom-in’ button on web pages, although this may not always pose a solution.
Performance Testing
Testing Strategy
To ensure the smooth operation of our bedside navigator with MotionInput, we conducted performance tests with fanless Intel NUCs, as this is the required installation environment.
Over a period of two hours, we collected data on the program's maximum RAM usage, maximum CPU usage, and the time it takes to load new pages.
- Intel MeLe Quieter 3Q
- Processor: Intel(R) Celeron(R) N5105 2.00GHz
- Installed RAM: 8.00 GB (7.75 GB usable)
- System type: 64-bit operating system, x64-based processor
Bedside Navigator Device 1
- Intel NUC Mini PC
- Processor: Intel(R) Core(TM) 17-8559U CPU @ 2.70GHz 2.71 GHz
- Installed RAM: 8.00 GB (7.87 GB usable)
- System type: 64-bit operating system, x64-based processor
Bedside Navigator Device 2
Results
Device | Maximum RAM Usage (MB) | Maximum CPU usage (%) | Loading time (sec) |
---|---|---|---|
Device 1 (Intel MeLe) | ~ 700 | ~ 33 | ~ 5 |
Device 2 (Intel NUC) | ~ 1015 | ~ 14 | ~ 2 |
For a device which will only be running our Bedside Navigator, the Maximum RAM Usage is acceptable. The user should not face major problems with the performance of the app whilst it is running. There will be minimal latency but should not be too noticeable. Although the NUC is fanless, there is no significant overheating.
User Acceptance Testing
Testing Strategy
We asked a total of four simulated testers, ranging from young to senior adults, to navigate FISECARE V2 using our MotionInput Bedside Navigator. In this way, we tested both the accuracy and comfortability of our MotionInput modes, as well as the user-friendliness of the interface.
Test Cases
Test Description | Success rate out of 4 (IN FACE MODE) | Average no. of attempts needed (IN FACE MODE) | Average usability/comfort rating out of 4 (IN FACE MODE) | Success rate out of 4 (IN HAND MODE) | Average no. of attempts needed (IN HAND MODE) | Average usability/comfort rating out of 4 (IN HAND MODE) |
---|---|---|---|---|---|---|
Zoom in and out of webpages on FISECARE | 3 | 2 | 3 | 4 | 1 | 3 |
Draw on Paint website | 3 | 2 | 2 | 3 | 1 | 3 |
Navigate to the prev page using the back button. | 3 | 1 | 4 | 4 | 1 | 4 |
Play scrabble. | 2 | 3 | 1 | 3 | 3 | 3 |
Find study materials for year 3 Maths | 4 | 1 | 3 | 4 | 1 | 4 |
Calculate simple arithmetic with the online calculator (e.g. 3 x 45) | 3 | 2 | 3 | 4 | 1 | 4 |
Play UNO with a friend | 3 | 2 | 3 | 4 | 2 | 3 |
Calibration steps are easy to follow | 3 | 2 | 4 | 4 | 2 | 4 |
The results of calibration are accurate and the user can navigate well | 3 | 1 | 3 | 4 | 2 | 3 |
Scrolling on pages | 3 | 2 | 2 | 4 | 2 | 3 |
- Laggy
- Accurate
- Moving as I want it e.g. speed
- Tiring on the hand
- Quite difficult to reach smaller buttons due to sensitivity but FISECARE is built with mainly big buttons so it does not pose a big negative impact on user experience.
Feedback for hand navigation:
- The mouse can be quite sensitive and hard to navigate to small buttons
- Sometimes when the user is acting out a gesture, the system does not respond. Other times, when the user is not acting out a gesture, the system may still respond causing unwanted clicking.
- Moving as I want it e.g. speed
- More information on how to use the joystick mode
Feedback for face navigation:
Results
Overall, similar to our results for unit testing we have found that hand mode provides larger control over the system. With unit testing and user acceptance testing combined, we have made improvements to our Bedside Navigator along the way. The main problems we faced by the face mode, and attempted to fix, include:
Jittering - even with the addition of the gaussian filter and other smoothing techniques, jittering has not been reduced. The root of the problem may be connected to the trembling nature of the MediaPipe Face Mesh model. Therefore, at calibration, the input is already considered bad. To improve, we will need to consider algorithms that can help make the model more stable.
Gestures - at extreme angles and with poor lighting, the gestures are rather sensitive or the gesture does not respond. This can be because of the gestures used to train the classifier which predicts which gesture the user is making, using training data from users facing the camera. However, with some further analysis, again the performance of our solution is bounded by the performance of the MediaPipe Face Mesh model.
Other careful improvements we have made along the way include:
improvements to the Calibration Window UI (explained in UI Design).
the default web apps available to the users on FISECARE - we wanted to choose web apps that were suitable to our target audience, free to use and easy to navigate with MotionInput considering the sensitivity of its gestures.
Improvements to the backend of calibration to improve jittering and reduce noise.
Attempt to improve gesture detection in some cases by manipulating the calculations that return whether the gesture is active or not.
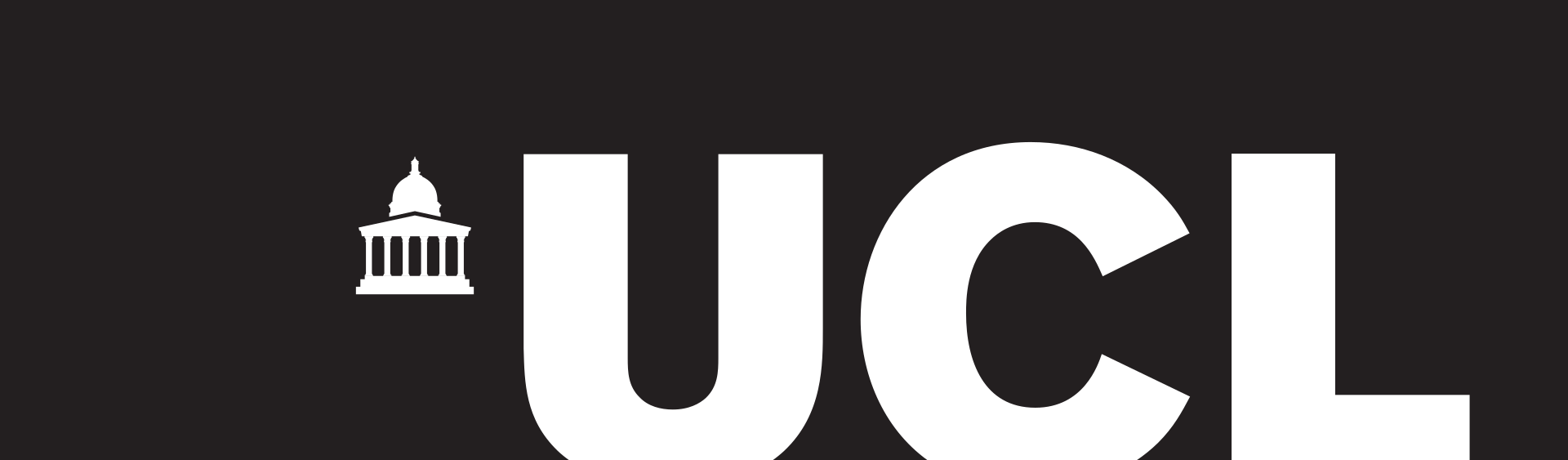
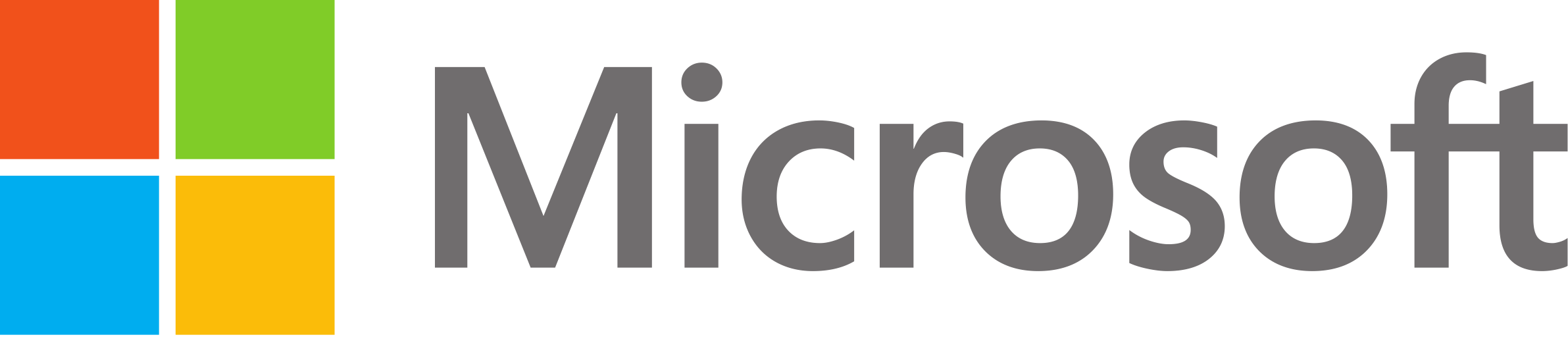

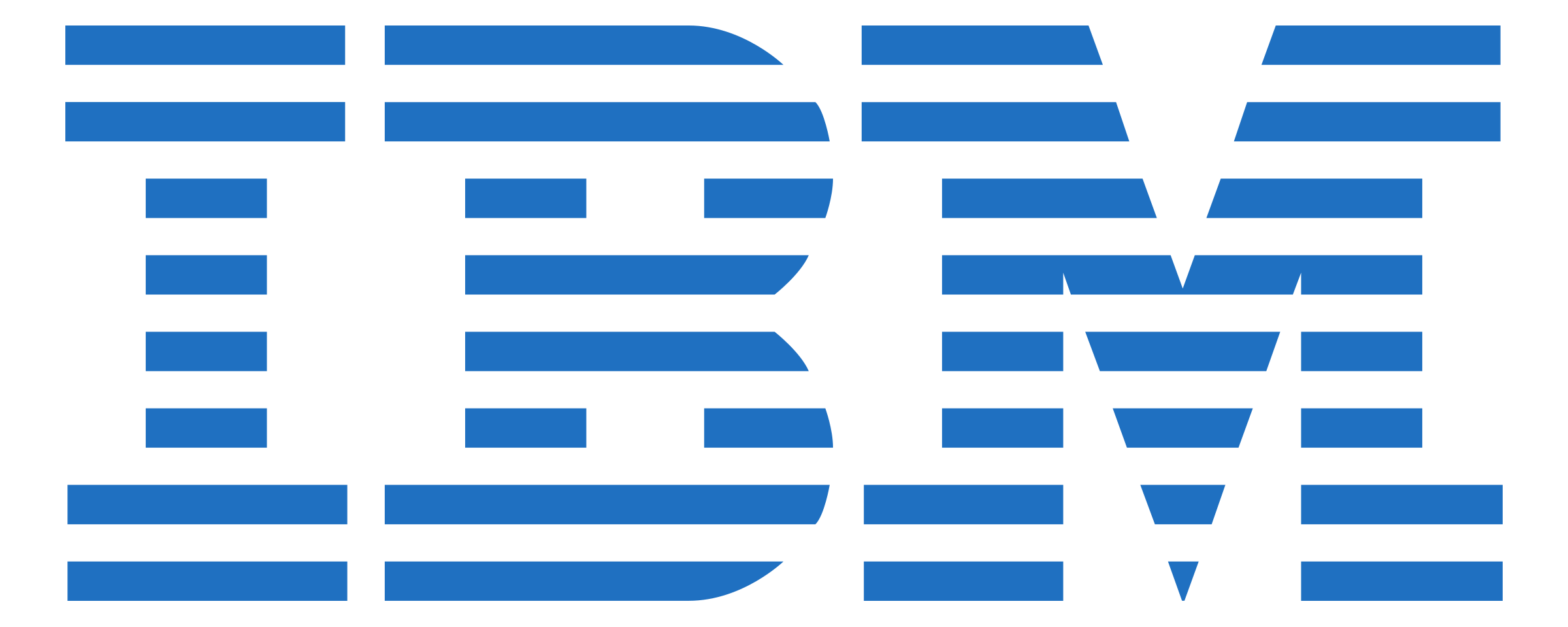