Technology Review
Depending on our needs, we have reviewed the following technologies:
Windows Presentation Foundation
For extending the FISECARE GUI, it makes sense to continue using the WPF framework that the previous team has chosen [1]. WPF provides a robust and modern framework that is able to run on Windows 11, and provides a variety of development features, which would make our lives easier. Additionally, the GUI will be coded using C#.
We needed to extend FISECARE to provide services for children rather than just the elderly. In order to do this, we researched educational website for kids and especially board games that they can play with friends and family at home to keep them connected and entertained. The websites that we chose are:
- Maths Games
- Revision
- Coding on Scratch
- Online Calculator
Educational
- Spelling Game
- Scrabble
- UNO
- Paint
Games and Creativity
-
Facial navigation
There are a number of facial recognition packages available in the field. In particular, we investigated the most popular ones: Dlib, MediaPipe, and similar models provided by OpenVINO’s Open Model Zoo.
MediaPipe provides two separate solutions for facial recognition: MediaPipe Face Mesh and Face Detection. Both solutions are proven to have sub-millisecond inference speed on mobile GPUs [3], which is essential for running on light-weight, fan-less devices. However, MediaPipe Face Mesh provides a much more accurate facial recognition, as it detects 468 landmarks [4], whereas MediaPipe Face Detect only detects only 6 [5].
Dlib was the initial choice made by MotionInput teams for facial recognition [6], with the reason that MediaPipe Face solutions were too slow. However this is not the case, as demonstrated by BlazeFace [3], as well as our testing cases. We asked Dlib and MediaPipe to detect face and display the nose-tip landmark on the screen, frame by frame. We discovered that both MediaPipe Face Mesh and Face Detect runs twice as fast as Dlib. Moreover, we discovered that Dlib is less accurate than both MediaPipe solutions.
OpenVINO is a toolkit that aims to optimise inference speed of machine learning models on different devices, by maximising usage of hardware resources [7]. It has supported a wide range of machine learning models, and we looked at those for facial recognition, as well as for head pose calculation. Specifically, we looked at Intel’s pre-trained models for facial recognition [8]. Although the models can detect landmarks for a fairly high accuracy, the output is in 2D coordinates.
-
Hand navigation
For hand detection, we looked at MediaPipe Hand module, which was the initial choice made by MotionInput teams for hand landmark detection [6], and is one of the most widely used models for hand tracking. It is able to detect 21 landmarks in 3D coordinates. The additional z-value provides the depth of the landmarks, which is essential to determining the movement of user’s hand (i.e. is it moving away or towards the camera).
-
Other Machine Learning Tool(s)
Additionally, we looked at the sklearn library, as we wanted to explore the potential of training a user-specific navigation model during the calibration stage of MotionInput. Sklearn provides a range of simple yet powerful models [9] which might be ideal for running MotionInput on small, fan-less computers.
Machine Learning
For extending MotionInput v3 face and hand navigation modules, since our main goal was to enable navigation from an angle, we looked at a range of Python computer vision libraries, as well as a few other machine learning libraries:Summary
-
In the end, our team decided on:
- WPF in C# for GUI
- MediaPipe Face Mesh for bedside facial navigation mode
- MediaPipe Hand for bedside hand navigation mode
- Sklearn for training user-specific navigation models
References
- [1] A. Niraula, P. Kamthornthip, and R. Silva, “Research,” FISECARE: Research, 2022. [Online]. Available: https://students.cs.ucl.ac.uk/2021/group35/research.html. [Accessed: 05-Mar-2023].
- [2] UCL Computer Science, “Touchless computing,” Touchless Computing at UCL Computer Science, 2022. [Online]. Available: https://www.touchlesscomputing.org/. [Accessed: 05-Mar-2023].
- [3] V. Bazarevsky, Y. Kartynnik, A. Vakunov, K. Raveendran, and M. Grundmann, “Blazeface: Sub-millisecond neural face detection on mobile gpus,” arXiv.org, 14-Jul-2019. [Online]. Available: https://arxiv.org/abs/1907.05047. [Accessed: 05-Mar-2023].
- [4] "MediaPipe Face Mesh," MediaPipe, Google LLC [Online]. Available: https://google.github.io/mediapipe/solutions/face_mesh.html. [Accessed: 24-Mar-2023]
- [5] "MediaPipe Face Detection," MediaPipe, Google LLC [Online]. Available: https://google.github.io/mediapipe/solutions/face_detection.html. [Accessed: 24-Mar-2023]
- [6] C. Meinson, J. Ho, and R. Bogdan, “Motioninput V3,” MotionInput V3 - Research, 2022. [Online]. Available: https://students.cs.ucl.ac.uk/2021/group32/research.html#mi2. [Accessed: 05-Mar-2023].
- [7] "OpenVINO Toolkit Documentation," Intel Corporation, 2023. [Online]. Available: https://docs.openvino.ai/latest/home.html. [Accessed: 24-Mar-2023]
- [8] "Object Recognition Models," OpenVINO Toolkit Documentation, Intel Corporation, 2023. [Online]. Available: https://docs.openvino.ai/latest/omz_models_group_intel.html#object-recognition-model. [Accessed: 24-Mar-2023]
- [9] F. Pedregosa et al., "Scikit-learn: Machine Learning in Python," Journal of Machine Learning Research, vol. 12, pp. 2825-2830, 2011.
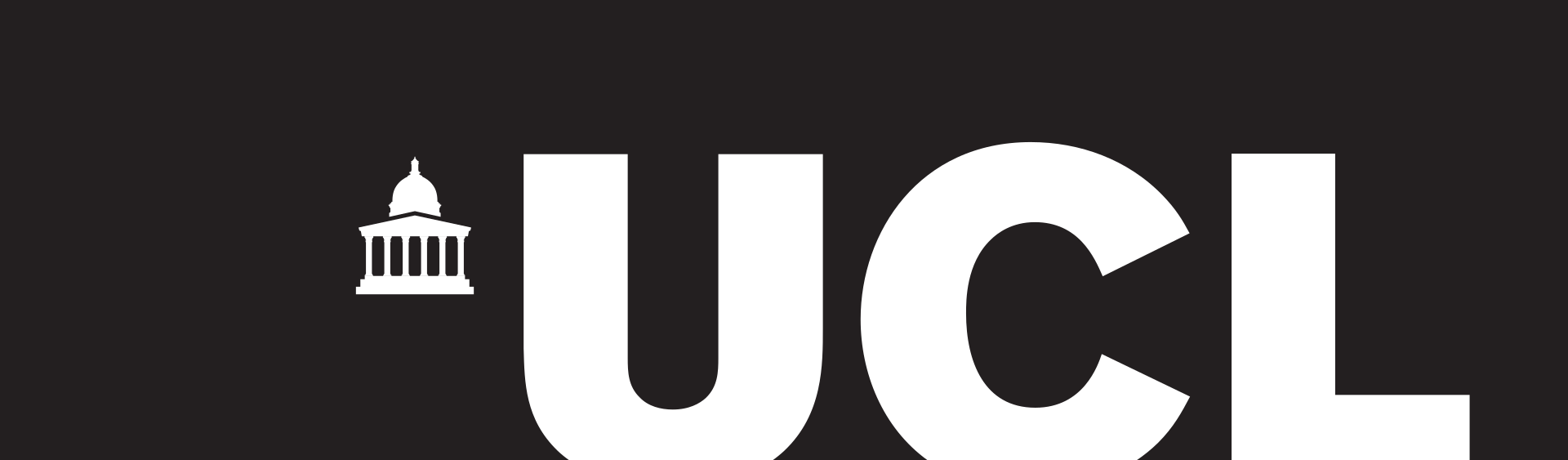
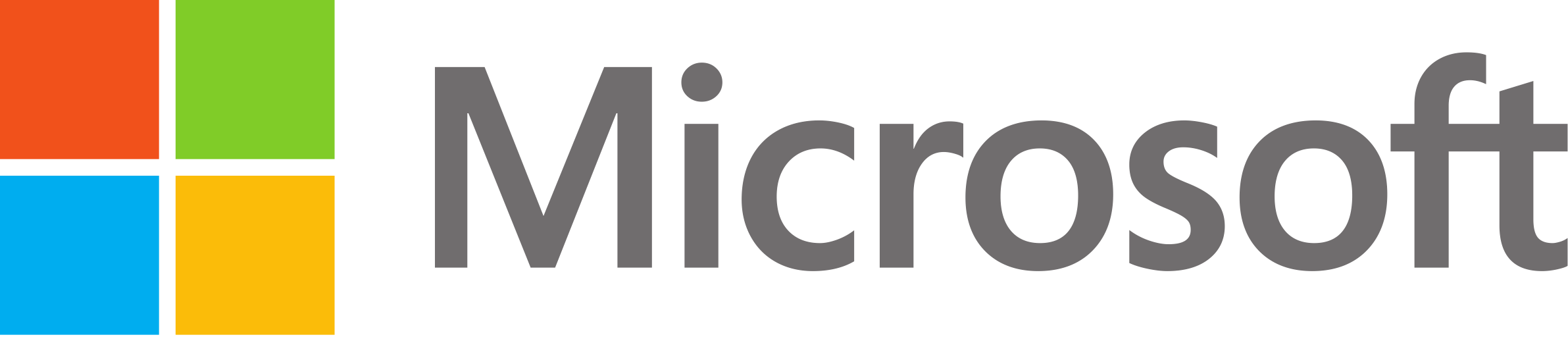

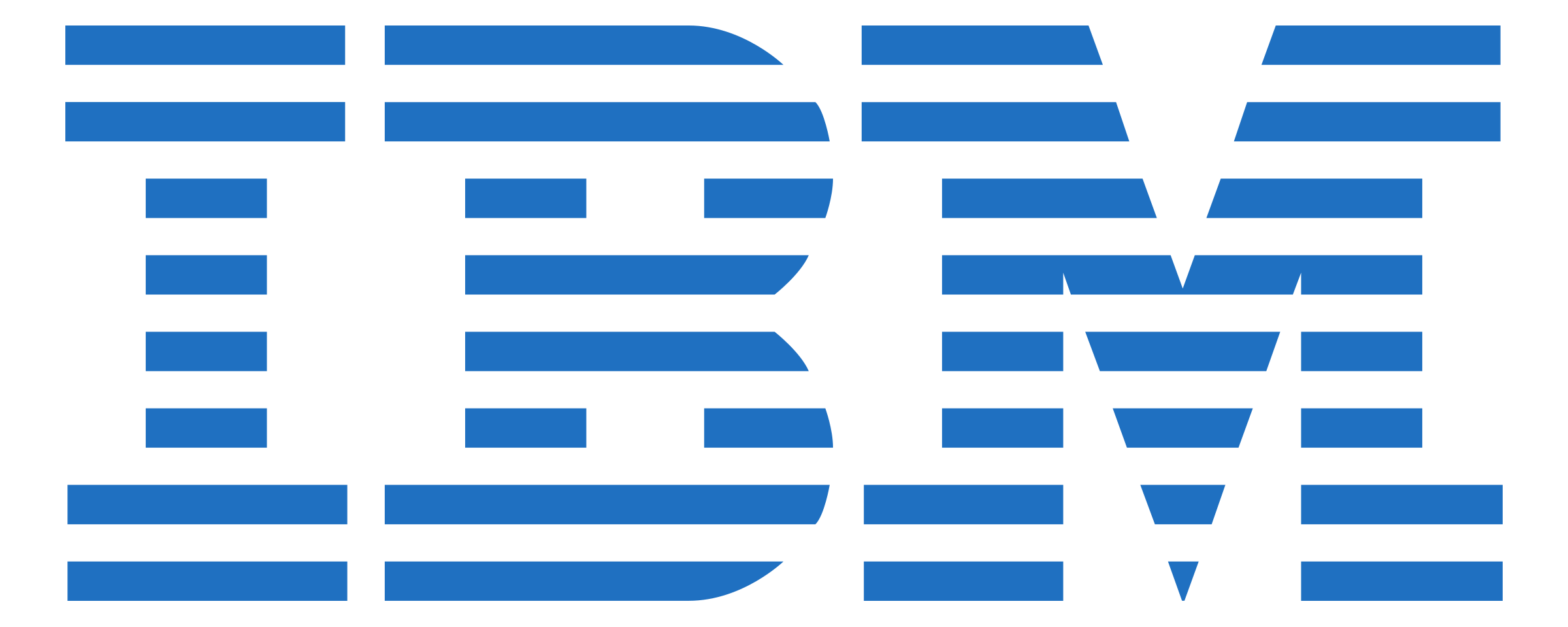