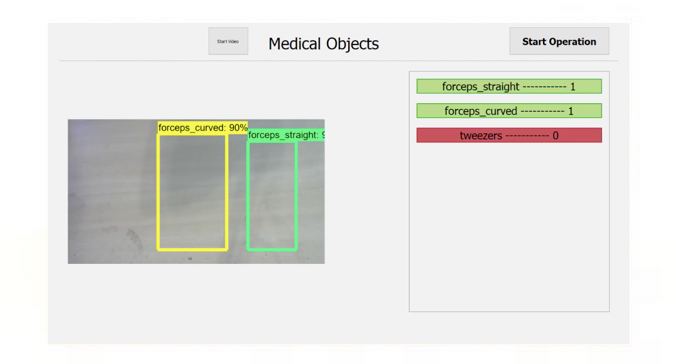
Computer Vision for object detection in Healthcare
See how tracking obejcts in operating rooms can make working easier
Learn More
How we use computer vision for object detection in healthcare
Working in an operating theatre is always nerve wracking and can be busy, leaving such an environment prone to making mistakes. With recent advances in Computer Visions, current technologies are able to identify vast numbers of objects in a wide variety of scenarios. By utilising such technologies, we can train machines to detect and identify different medical instruments used in clinical environments.
We wanted to create a system that allows doctors and nurse practitioners to monitor instruments being used in their operating theatres. The system will be able to display what instruments are present in the operation tray and correctly identify what and when instruments are currently in use when they are removed. Thus allowing all staff to gain a clearer overview of the operation at any given time.
By gathering all this data, complete operation summaries and timelines can be produced. All of this real world data can be analysed and shared allowing for surgeons, junior doctors and medical students alike to improve and learn. We hope our system will open up avenues in the medical field and hopefully allow the field to advance further.
By having a simple user interface we allow the staff to understand and process information more easily.
Using information gathered by the system we will be able to gain valuable insight into how medical instruments are handled.
Object Detection calculations are made on the cloud allowing access to our API from any machine and faster detections.
The people behind the magic
Lead Programmer, Researcher & Client Liason
benedict.chan.17@ucl.ac.ukClient Liason,UI Designer, Website Designer & Programmer
shirin.harandi.17@ucl.ac.ukBi-weekly reports on what the team did during that time. All the reports can be viewed here.